|
Big Data Business Intelligence for Criminal Intelligence Analysis培训
|
|
班级规模及环境--热线:4008699035 手机:15921673576( 微信同号) |
每个班级的人数限3到5人,互动授课, 保障效果,小班授课。 |
上间和地点 |
上课地点:【上海】:同济大学(沪西)/新城金郡商务楼(11号线白银路站) 【深圳分部】:电影大厦(地铁一号线大剧院站)/深圳大学成教院 【北京分部】:北京中山学院/福鑫大楼 【南京分部】:金港大厦(和燕路) 【武汉分部】:佳源大厦(高新二路) 【成都分部】:领馆区1号(中和大道) 【沈阳分部】:沈阳理工大学/六宅臻品 【郑州分部】:郑州大学/锦华大厦 【石家庄分部】:河北科技大学/瑞景大厦 【广州分部】:广粮大厦 【西安分部】:协同大厦 最近开间(周末班/连续班/晚班):2018年3月18日 |
实验设备 |
◆小班教学,教学效果好 ☆注重质量☆边讲边练 ☆合格学员免费推荐工作 ★实验设备请点击这儿查看★ |
质量保障 |
1、培训过程中,如有部分内容理解不透或消化不好,可免费在以后培训班中重听; 2、培训结束后,授课老师留给学员联系方式,保障培训效果,免费提供课后技术支持。 3、培训合格学员可享受免费推荐就业机会。☆合格学员免费颁发相关工程师等资格证书,提升职业资质。专注高端技术培训15年,曙海学员的能力得到大家的认同,受到用人单位的广泛赞誉,曙海的证书受到广泛认可。 |
课程大纲 |
|
- Day 01
=====
Overview of Big Data Business Intelligence for Criminal Intelligence Analysis
- Case Studies from Law Enforcement - Predictive Policing
Big Data adoption rate in Law Enforcement Agencies and how they are aligning their future operation around Big Data Predictive Analytics
Emerging technology solutions such as gunshot sensors, surveillance video and social media
Using Big Data technology to mitigate information overload
Interfacing Big Data with Legacy data
Basic understanding of enabling technologies in predictive analytics
Data Integration & Dashboard visualization
Fraud management
Business Rules and Fraud detection
Threat detection and profiling
Cost benefit analysis for Big Data implementation
Introduction to Big Data
- Main characteristics of Big Data -- Volume, Variety, Velocity and Veracity.
MPP (Massively Parallel Processing) architecture
Data Warehouses – static schema, slowly evolving dataset
MPP Databases: Greenplum, Exadata, Teradata, Netezza, Vertica etc.
Hadoop Based Solutions – no conditions on structure of dataset.
Typical pattern : HDFS, MapReduce (crunch), retrieve from HDFS
Apache Spark for stream processing
Batch- suited for analytical/non-interactive
Volume : CEP streaming data
Typical choices – CEP products (e.g. Infostreams, Apama, MarkLogic etc)
Less production ready – Storm/S4
NoSQL Databases – (columnar and key-value): Best suited as analytical adjunct to data warehouse/database
NoSQL solutions
- KV Store - Keyspace, Flare, SchemaFree, RAMCloud, Oracle NoSQL Database (OnDB)
KV Store - Dynamo, Voldemort, Dynomite, SubRecord, Mo8onDb, DovetailDB
KV Store (Hierarchical) - GT.m, Cache
KV Store (Ordered) - TokyoTyrant, Lightcloud, NMDB, Luxio, MemcacheDB, Actord
KV Cache - Memcached, Repcached, Coherence, Infinispan, EXtremeScale, JBossCache, Velocity, Terracoqua
Tuple Store - Gigaspaces, Coord, Apache River
Object Database - ZopeDB, DB40, Shoal
Document Store - CouchDB, Cloudant, Couchbase, MongoDB, Jackrabbit, XML-Databases, ThruDB, CloudKit, Prsevere, Riak-Basho, Scalaris
Wide Columnar Store - BigTable, HBase, Apache Cassandra, Hypertable, KAI, OpenNeptune, Qbase, KDI
Varieties of Data: Introduction to Data Cleaning issues in Big Data
- RDBMS – static structure/schema, does not promote agile, exploratory environment.
NoSQL – semi structured, enough structure to store data without exact schema before storing data
Data cleaning issues
Hadoop
- When to select Hadoop?
STRUCTURED - Enterprise data warehouses/databases can store massive data (at a cost) but impose structure (not good for active exploration)
SEMI STRUCTURED data – difficult to carry out using traditional solutions (DW/DB)
Warehousing data = HUGE effort and static even after implementation
For variety & volume of data, crunched on commodity hardware – HADOOP
Commodity H/W needed to create a Hadoop Cluster
Introduction to Map Reduce /HDFS
- MapReduce – distribute computing over multiple servers
HDFS – make data available locally for the computing process (with redundancy)
Data – can be unstructured/schema-less (unlike RDBMS)
Developer responsibility to make sense of data
Programming MapReduce = working with Java (pros/cons), manually loading data into HDFS
=====
Day 02
=====
Big Data Ecosystem -- Building Big Data ETL (Extract, Transform, Load) -- Which Big Data Tools to use and when?
- Hadoop vs. Other NoSQL solutions
For interactive, random access to data
Hbase (column oriented database) on top of Hadoop
Random access to data but restrictions imposed (max 1 PB)
Not good for ad-hoc analytics, good for logging, counting, time-series
Sqoop - Import from databases to Hive or HDFS (JDBC/ODBC access)
Flume – Stream data (e.g. log data) into HDFS
Big Data Management System
- Moving parts, compute nodes start/fail :ZooKeeper - For configuration/coordination/naming services
Complex pipeline/workflow: Oozie – manage workflow, dependencies, daisy chain
Deploy, configure, cluster management, upgrade etc (sys admin) :Ambari
In Cloud : Whirr
Predictive Analytics -- Fundamental Techniques and Machine Learning based Business Intelligence
- Introduction to Machine Learning
Learning classification techniques
Bayesian Prediction -- preparing a training file
Support Vector Machine
KNN p-Tree Algebra & vertical mining
Neural Networks
Big Data large variable problem -- Random forest (RF)
Big Data Automation problem – Multi-model ensemble RF
Automation through Soft10-M
Text analytic tool-Treeminer
Agile learning
Agent based learning
Distributed learning
Introduction to Open source Tools for predictive analytics : R, Python, Rapidminer, Mahut
Predictive Analytics Ecosystem and its application in Criminal Intelligence Analysis
- Technology and the investigative process
Insight analytic
Visualization analytics
Structured predictive analytics
Unstructured predictive analytics
Threat/fraudstar/vendor profiling
Recommendation Engine
Pattern detection
Rule/Scenario discovery – failure, fraud, optimization
Root cause discovery
Sentiment analysis
CRM analytics
Network analytics
Text analytics for obtaining insights from transcripts, witness statements, internet chatter, etc.
Technology assisted review
Fraud analytics
Real Time Analytic
=====
Day 03
=====
Real Time and Scalable Analytics Over Hadoop
- Why common analytic algorithms fail in Hadoop/HDFS
Apache Hama- for Bulk Synchronous distributed computing
Apache SPARK- for cluster computing and real time analytic
CMU Graphics Lab2- Graph based asynchronous approach to distributed computing
KNN p -- Algebra based approach from Treeminer for reduced hardware cost of operation
Tools for eDiscovery and Forensics
- eDiscovery over Big Data vs. Legacy data – a comparison of cost and performance
Predictive coding and Technology Assisted Review (TAR)
Live demo of vMiner for understanding how TAR enables faster discovery
Faster indexing through HDFS – Velocity of data
NLP (Natural Language processing) – open source products and techniques
eDiscovery in foreign languages -- technology for foreign language processing
Big Data BI for Cyber Security – Getting a 360-degree view, speedy data collection and threat identification
- Understanding the basics of security analytics -- attack surface, security misconfiguration, host defenses
Network infrastructure / Large datapipe / Response ETL for real time analytic
Prescriptive vs predictive – Fixed rule based vs auto-discovery of threat rules from Meta data
Gathering disparate data for Criminal Intelligence Analysis
- Using IoT (Internet of Things) as sensors for capturing data
Using Satellite Imagery for Domestic Surveillance
Using surveillance and image data for criminal identification
Other data gathering technologies -- drones, body cameras, GPS tagging systems and thermal imaging technology
Combining automated data retrieval with data obtained from informants, interrogation, and research
Forecasting criminal activity
=====
Day 04
=====
Fraud prevention BI from Big Data in Fraud Analytics
- Basic classification of Fraud Analytics -- rules-based vs predictive analytics
Supervised vs unsupervised Machine learning for Fraud pattern detection
Business to business fraud, medical claims fraud, insurance fraud, tax evasion and money laundering
Social Media Analytics -- Intelligence gathering and analysis
- How Social Media is used by criminals to organize, recruit and plan
Big Data ETL API for extracting social media data
Text, image, meta data and video
Sentiment analysis from social media feed
Contextual and non-contextual filtering of social media feed
Social Media Dashboard to integrate diverse social media
Automated profiling of social media profile
Live demo of each analytic will be given through Treeminer Tool
Big Data Analytics in image processing and video feeds
- Image Storage techniques in Big Data -- Storage solution for data exceeding petabytes
LTFS (Linear Tape File System) and LTO (Linear Tape Open)
GPFS-LTFS (General Parallel File System - Linear Tape File System) -- layered storage solution for Big image data
Fundamentals of image analytics
Object recognition
Image segmentation
Motion tracking
3-D image reconstruction
Biometrics, DNA and Next Generation Identification Programs
- Beyond fingerprinting and facial recognition
Speech recognition, keystroke (analyzing a users typing pattern) and CODIS (combined DNA Index System)
Beyond DNA matching: using forensic DNA phenotyping to construct a face from DNA samples
Big Data Dashboard for quick accessibility of diverse data and display :
- Integration of existing application platform with Big Data Dashboard
Big Data management
Case Study of Big Data Dashboard: Tableau and Pentaho
Use Big Data app to push location based services in Govt.
Tracking system and management
=====
Day 05
=====
How to justify Big Data BI implementation within an organization:
- Defining the ROI (Return on Investment) for implementing Big Data
Case studies for saving Analyst Time in collection and preparation of Data – increasing productivity
Revenue gain from lower database licensing cost
Revenue gain from location based services
Cost savings from fraud prevention
An integrated spreadsheet approach for calculating approximate expenses vs. Revenue gain/savings from Big Data implementation.
Step by Step procedure for replacing a legacy data system with a Big Data System
- Big Data Migration Roadmap
What critical information is needed before architecting a Big Data system?
What are the different ways for calculating Volume, Velocity, Variety and Veracity of data
How to estimate data growth
Case studies
Review of Big Data Vendors and review of their products.
- Accenture
APTEAN (Formerly CDC Software)
Cisco Systems
Cloudera
Dell
EMC
GoodData Corporation
Guavus
Hitachi Data Systems
Hortonworks
HP
IBM
Informatica
Intel
Jaspersoft
Microsoft
MongoDB (Formerly 10Gen)
MU Sigma
Netapp
Opera Solutions
Oracle
Pentaho
Platfora
Qliktech
Quantum
Rackspace
Revolution Analytics
Salesforce
SAP
SAS Institute
Sisense
Software AG/Terracotta
Soft10 Automation
Splunk
Sqrrl
Supermicro
Tableau Software
Teradata
Think Big Analytics
Tidemark Systems
Treeminer
VMware (Part of EMC)
Q/A session
|
|
|
|
|
|
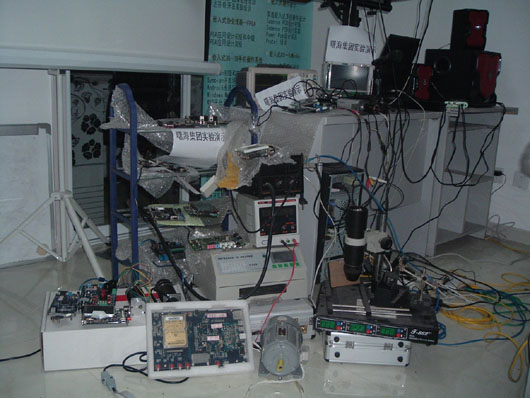 * |
|
本部份程部分实验室实景 |
|
|
|
|
|
|
合作伙伴与授权机构 |
 Altera全球合作培训机构 |
 诺基亚Symbian公司授权培训中心 |
 Atmel公司全球战略合作伙伴 |
 微软全球嵌入式培训合作伙伴 |
 英国ARM公司授权培训中心 |
 ARM工具关键合作单位 |
|
|
|
我们培训过的企业客户评价: |
曙海的andriod系统与应用培训完全符合了我公司的要求,达到了我公司培训的目的。特别值得一提的是授部份讲师针对我们公司的开发的项目专门提供了一些很好程序的源代码,基本满足了我们的项目要求。 ——上海贝尔,李工 曙海培训DSP2000的老师,上部份思路清晰,口齿清楚,由浅入深,重点突出,培训效果是不错的, 达到了我们想要的效果,希望继续合作下去。 ——中国电子科技集团技术部主任马工 曙海的FPGA培训很好地填补了高校FPGA培训空白,不错。总之,有利于学生的发展,有利于教师的发展,有利于部份程的发展,有利于社会的发展。 ——上海电子学院,冯老师 曙海给我们公司提供的Dsp6000培训,符合我们项目的开发要求,解决了很多困惑我们很久的问题,与曙海的合作非常愉快。 ——公安部第三研究所,项目部负责人李先生 MTK培训-我在网上找了很久,就是找不到。在曙海居然有MTK驱动的培训,老师经验很丰富,知识面很广。下一个还想培训IPHONE苹果手机。跟他们合作很愉快,老师很有人情味,态度很和蔼。 ——台湾双扬科技,研发处经理,杨先生 曙海对我们公司的iPhone培训,实验项目很多,确实学到了东西。受益无穷啊!特别是对于那种正在开发项目的,确实是物超所值。 ——台湾欧泽科技,张工 通过参加Symbian培训,再做Symbian相关的项目感觉更加得心应手了,理论加实践的授部份方式,很有针对性,非常的适合我们。学完之后,很轻松的就完成了我们的项目。 ——IBM公司,沈经理 有曙海这样的DSP开发培训单位,是教育行业的财富,听了他们的部份,茅塞顿开。 ——上海医疗器械高等学校,罗老师
|
我们最新培训过的企业客户以及培训的主要内容: |
|
一汽海马汽车DSP培训 苏州金属研究院DSP培训 南京南瑞集团技术FPGA培训 西安爱生技术集团FPGA培训,DSP培训 成都熊谷加世电气DSP培训 福斯赛诺分析仪器(苏州)FPGA培训 南京国电工程FPGA培训 北京环境特性研究所达芬奇培训 中国科学院微系统与信息技术研究所FPGA高级培训 重庆网视只能流技术开发达芬奇培训 无锡力芯微电子股份IC电磁兼容 河北科学院研究所FPGA培训 上海微小卫星工程中心DSP培训 广州航天航空POWERPC培训 桂林航天工学院DSP培训 江苏五维电子科技达芬奇培训 无锡步进电机自动控制技术DSP培训 江门市安利电源工程DSP培训 长江力伟股份CADENCE培训 爱普生科技(无锡)数字模拟电路 河南平高电气DSP培训 中国航天员科研训练中心A/D仿真 常州易控汽车电子WINDOWS驱动培训 南通大学DSP培训 上海集成电路研发中心达芬奇培训 北京瑞志合众科技WINDOWS驱动培训 江苏金智科技股份FPGA高级培训 中国重工第710研究所FPGA高级培训 芜湖伯特利汽车安全系统DSP培训 厦门中智能软件技术Android培训 上海科慢车辆部件系统EMC培训 中国电子科技集团第五十研究所,软件无线电培训 苏州浩克系统科技FPGA培训 上海申达自动防范系统FPGA培训 四川长虹佳华信息MTK培训 公安部第三研究所--FPGA初中高技术开发培训以及DSP达芬奇芯片视频、图像处理技术培训 上海电子信息职业技术学院--FPGA高级开发技术培训 上海点逸网络科技有限公司--3G手机ANDROID应用和系统开发技术培训 格科微电子有限公司--MTK应用(MMI)和驱动开发技术培训 南昌航空大学--fpga高级开发技术培训 IBM公司--3G手机ANDROID系统和应用技术开发培训 上海贝尔--3G手机ANDROID系统和应用技术开发培训 中国双飞--Vxworks应用和BSP开发技术培训
|
上海水务建设工程有限公司--Alter/XilinxFPGA应用开发技术培训 恩法半导体科技--AllegroCandencePCB仿真和信号完整性技术培训 中国计量学院--3G手机ANDROID应用和系统开发技术培训 冠捷科技--FPGA芯片设计技术培训 芬尼克兹节能设备--FPGA高级技术开发培训 川奇光电--3G手机ANDROID系统和应用技术开发培训 东华大学--Dsp6000系统开发技术培训 上海理工大学--FPGA高级开发技术培训 同济大学--Dsp6000图像/视频处理技术培训 上海医疗器械高等专科学校--Dsp6000图像/视频处理技术培训 中航工业无线电电子研究所--Vxworks应用和BSP开发技术培训 北京交通大学--Powerpc开发技术培训 浙江理工大学--Dsp6000图像/视频处理技术培训 台湾双阳科技股份有限公司--MTK应用(MMI)和驱动开发技术培训 滚石移动--MTK应用(MMI)和驱动开发技术培训 冠捷半导体--Linux系统开发技术培训 奥波--CortexM3+uC/OS开发技术培训 迅时通信--WinCE应用与驱动开发技术培训 海鹰医疗电子系统--DSP6000图像处理技术培训 博耀科技--Linux系统开发技术培训 华路时代信息技术--VxWorksBSP开发技术培训 台湾欧泽科技--iPhone开发技术培训 宝康电子--AllegroCandencePCB仿真和信号完整性技术培训 上海天能电子有限公司--AllegroCandencePCB仿真和信号完整性技术培训 上海亨通光电科技有限公司--andriod应用和系统移植技术培训 上海智搜文化传播有限公司--Symbian开发培训 先先信息科技有限公司--brew手机开发技术培训 鼎捷集团--MTK应用(MMI)和驱动开发技术培训 傲然科技--MTK应用(MMI)和驱动开发技术培训 中软国际--Linux系统开发技术培训 龙旗控股集团--MTK应用(MMI)和驱动开发技术培训 研祥智能股份有限公司--MTK应用(MMI)和驱动开发技术培训 罗氏诊断--Linux应用开发技术培训 西东控制集团--DSP2000应用技术及DSP2000在光伏并网发电中的应用与开发 科大讯飞--MTK应用(MMI)和驱动开发技术培训 东北农业大学--IPHONE苹果应用开发技术培训 中国电子科技集团--Dsp2000系统和应用开发技术培训 中国船舶重工集团--Dsp2000系统开发技术培训 晶方半导体--FPGA初中高技术培训 肯特智能仪器有限公司--FPGA初中高技术培训 哈尔滨大学--IPHONE苹果应用开发技术培训 昆明电器科学研究所--Dsp2000系统开发技术 奇瑞汽车股份--单片机应用开发技术培训
|
|
|
|
|